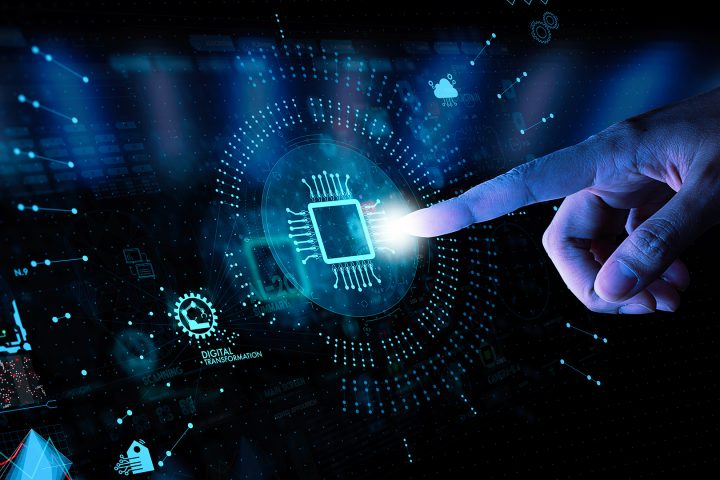
AI is transforming how businesses operate, but for many IBM i users, the path forward feels unclear. Questions pile up: Where does one begin? How do you choose a project that delivers real results? What if AI causes more disruption than benefit?
The challenge is real: large-scale AI projects demand significant time, resources, and expertise. There is new terminology, new skills, and a blizzard of possible tools. For most organizations, this feels out of reach. But the solution lies in starting small.
Small AI projects solve specific problems, provide value, and build confidence without overhauls or unreasonable risk. Learn how IBM i organizations can use focused AI projects to solve critical challenges, build momentum, and create confidence for long-term AI success.
Small AI Projects Deliver Quick Wins
The smartest way to start is to focus on one specific problem and apply AI to solve it. Traditionally, IBM I databases hold a wealth of data—transaction records, inventory patterns, and customer behavior—that businesses can leverage without system overhaul. Small projects make use of this data and reveal value in incremental steps. A clear and manageable scope helps businesses minimize complexity and deliver measurable outcomes.
Small projects succeed because they answer immediate questions. For example, manufacturers often face challenges with demand forecasting, which leads to overproduction or stockouts. AI models built with historical data can help forecast production needs with accuracy.
For another example, think about companies that manage large internal document repositories. AI can enable these companies to structure data into searchable, efficient systems that save time and boosts productivity. These solutions address immediate challenges and demonstrate tangible value.
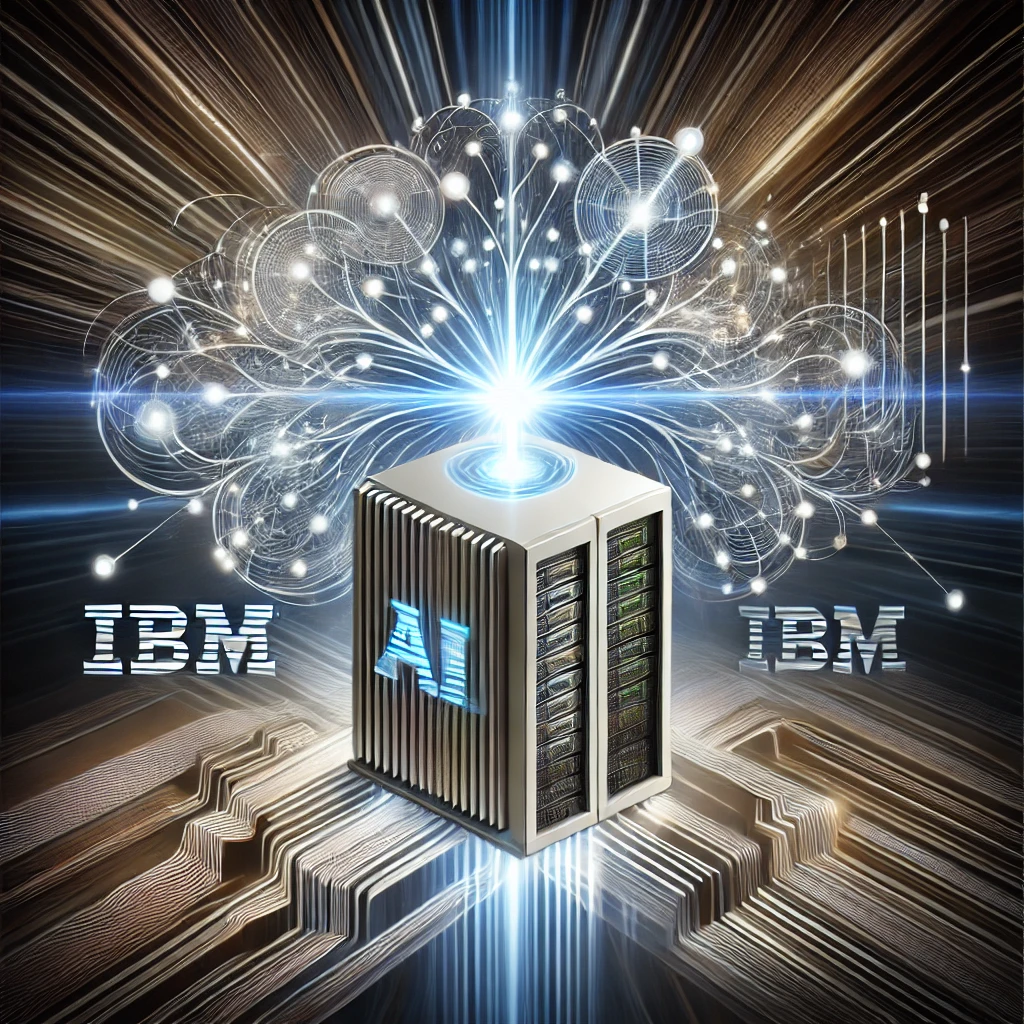
Results build trust in AI’s potential. As businesses see improvements—fewer stockouts, faster document access, or better decisions—they gain confidence to explore additional use cases. These early wins create buy-in from leadership and teams. Successes show the organization how AI augments the existing systems and increases the ability to recognize future use of AI to bring new efficiency.
IBM i environments already have an edge. Their systems are stable, their data is reliable, and their operations are proven. Targeted AI projects built on this foundation create an opportunity to explore innovation with low risk and high potential. It gives businesses a chance to test AI, reduce risks, and create value. The aim is not to disrupt what already works but to enhance it.
Every AI Project Needs a Data Scientist
AI projects succeed or fail based on one core factor: the quality and usability of data. Raw data on IBM i systems does not translate to value on its own. A data scientist bridges this gap. They help refine data into an asset that serves clear business objectives, and ensure AI delivers practical, measurable outcomes.
A data scientist helps businesses cut through the noise of off-the-shelf AI tools that promise plug-and-play simplicity but often fail to address real needs. They guide decisions, ensure AI tools integrate with IBM i environments and solve meaningful problems. For smaller projects, this guidance prevents missteps and ensures resources are spent efficiently.
Data scientists also provide clarity and confidence, especially for teams that have not undertaken AI projects in the past. They simplify complex processes and introduce practical applications like document optimization or demand forecast that fit seamlessly into current workflows. Focused, result-oriented projects led by data scientists build a foundation of trust in AI.
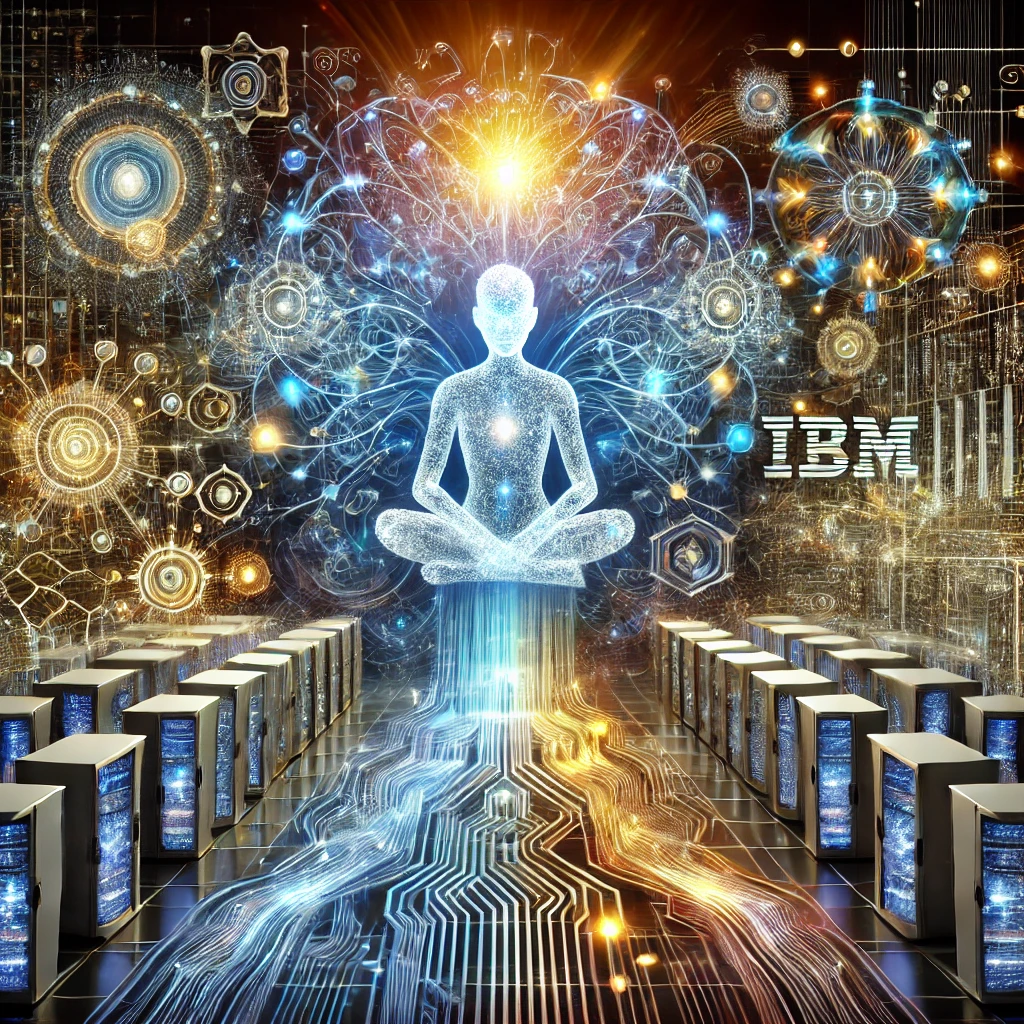
Perhaps their most significant contribution lies in preparing organizations for the future. A data scientist not only solves immediate challenges but equips teams to manage and scale AI over time. For IBM i organizations, this approach can help a business carefully navigate from an environment without AI to a new perspective that judiciously applies AI where it may best add value to the business.
Hiring a full-time data scientist is not feasible for most organizations. A better option is to work with a partner that offers access to skilled data science resources as needed. Fresche offers tailored discovery services and data science expertise.
The flexible model allows businesses to access the right skills at the right time, aligning AI initiatives with immediate objectives and long-term strategies. This partnership ensures AI becomes accessible and impactful with manageable costs.
Small Projects Make AI Challenges Manageable
AI projects bring challenges that require businesses to rethink their strategies, and IBM i environments are no exception. The first challenge is the volume of AI tools available in the marketplace. Many claim to deliver instant results but fail to address the unique requirements of IBM i systems.
Businesses cannot afford to choose tools based on promises alone. They must focus on solutions that align with their specific needs, integrate seamlessly with current systems, and provide measurable outcomes. Careful evaluation ensures every investment serves a clear purpose.
Another challenge lies in how businesses manage time, money, and expertise. Even small AI initiatives require structured tests, refinements, and adjustments to meet goals and deliver more reliable outcomes.
The key to success is defining precise goals and executing in achievable phases. This strategy ensures steady progress, educates resources, and strengthens confidence with each completed milestone.
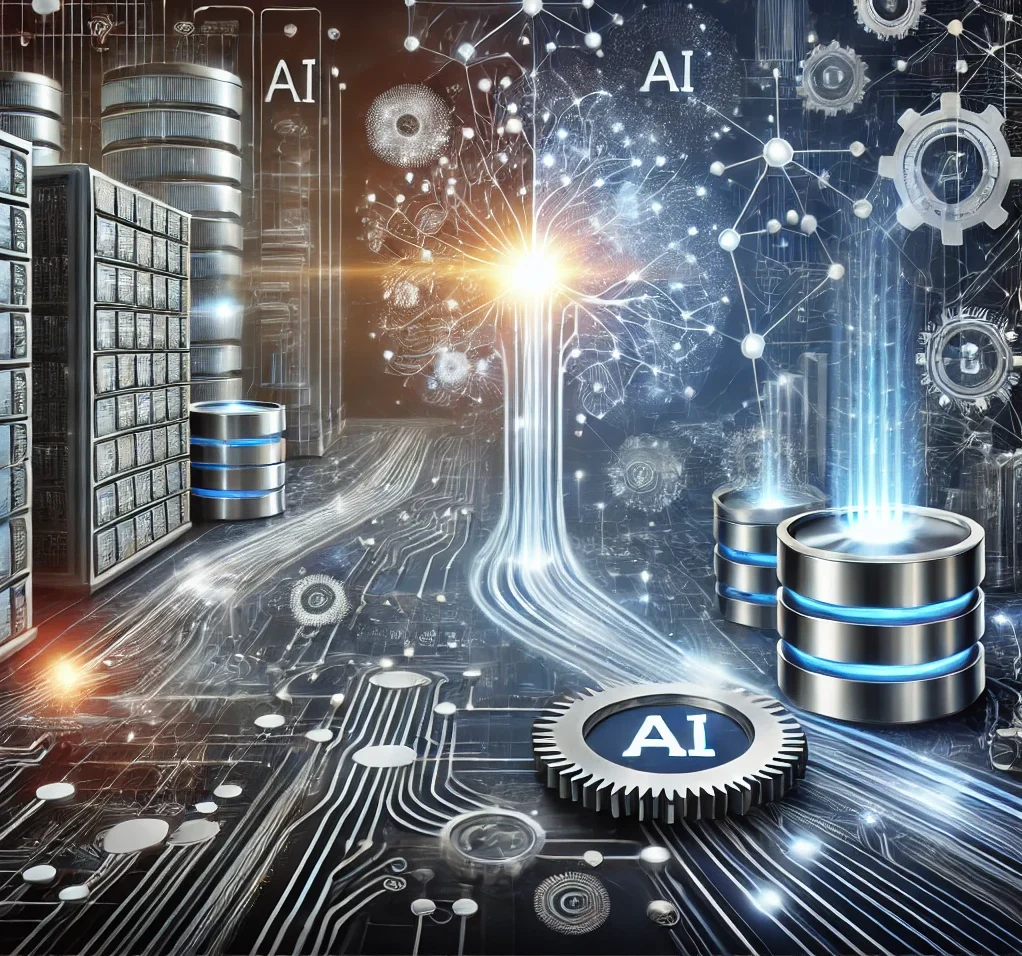
IBM i environments add another layer of complexity. These systems excel at stability, but they require a thoughtful approach to avoid disruption. The best strategy is to begin with small projects that enhance rather than replace. For instance, use AI to analyze historical customer support tickets and identify repetitive issues.
Another idea is to leverage AI for sales data analysis to recommend personalized offers for customers, increase conversion rates and loyalty without altering the existing sales infrastructure. Similarly, AI can detect patterns in payment errors to enable faster resolutions and minimize delays.
Finally, AI evolves rapidly. Models, tools, and strategies need regular updates to stay current. Organizations must embrace adaptability as part of their AI strategy. They must remain aware of advances in AI capability and select solutions that align with future company goals.
Want to explore how to develop a winning AI strategy? Download our whitepaper – Enterprise AI for IBM i: Craft an AI Transformation Strategy for Growth.
Choose the First AI Project
A well-chosen AI project focuses on a specific problem that affects day-to-day operations and offers measurable results within a short timeframe. Consider tasks that rely heavily on manual processes but provide little strategic value.
For example, automation of routine inventory checks or sales forecast generation from historical data saves time and improves accuracy. These projects eliminate inefficiencies and provide insights that teams can act on.
The data richness of IBM i systems provides another advantage. With years of operational data, businesses can develop predictive models or streamline workflows. A first AI project could focus on patterns in customer purchases or production cycles to refine demand forecasts. Choose a project that produces tangible results and educates the organization on how AI fits within their ecosystem.
Need more clarity on where to begin with AI? Fresche’s AI-Celerate helps IBM i organizations uncover high-impact opportunities tailored to business goals. This program evaluates your data, defines strategic priorities, and identifies projects that offer the highest ROI. Book a free AI consultation today to get started.
Common Misconceptions About AI
There are many misconceptions about AI adoption that can prevent businesses from taking the first step. A common myth is the belief that AI works straight out of the box. This idea sets businesses up for frustration when tools fail to deliver results.
AI is not a shortcut—it’s a strategic initiative. It requires clear goals, proper data preparation, and a focus on how it enhances existing systems. Companies that approach AI with a plan for integration and refinement see it as a tool for growth, not an off-the-shelf solution.
There is also a misconception that small projects lack impact. Many assume that meaningful AI initiatives require large-scale efforts to deliver value. Small, targeted projects are impactful because they focus on specific, high-priority challenges. For example, automation of a single manual task can lead to measurable gains.
Another myth is that small AI projects are just tests with no real-world relevance. Businesses often see these initiatives as practice runs rather than solutions that address actual problems. This view underestimates the potential to start AI implementation with a focused and productive scope. These early wins are not placeholders—they lay the foundation to scale AI intelligently.
Some believe that small AI projects still require significant upfront investment. The reality is different. Small projects focus on specific use cases and avoid unnecessary resource demands or infrastructure overhauls. Businesses can often use current tools and data to achieve results without significant investments.
Build Momentum Through Small Wins
The road to AI success begins with a simple decision: take the first step. These targeted AI projects establish a foundation to help teams and leadership see AI as a tool for progress, not disruption. With each success, businesses gain motivation and the understanding of how to identify where AI can best be employed in new initiatives to bring value to the business.
Each incremental step builds momentum and equips teams with knowledge, processes, and confidence to scale AI across the organization. For IBM i organizations, it is an opportunity to redefine what’s possible and lead in the age of AI.